Upcoming/ongoing
2024 - 2026
Dutch, More or Less. Contemporary Architecture, Design and Digital Culture
2024
2024
2024
2024
2024
Workshop
2024
2024
2025
Artificial vs. Intelligence
About me
I am a researcher, artist and engineer with an academic background counting degrees in Physics, Electrical Engineering, Neuropsychology, Artificial Intelligence and ArtScience. My professional history includes leading a machine learning team specializing in speech-to-intent systems and hands-on experience as both a researcher and an engineer in the field of generative AI.
In my artistic endeavors I explore the intersection of technology and life, creating interactive installations that invite reflection on the essence of being. My projects often endow things with a spark of life, challenging perceptions of existence. My approach is characterized by playful engagement with artificial intelligence, seeking to emulate the behaviors of living beings in a way that resonates with and surprises both myself and my audience, blurring the lines between the animate and the inanimate.
Works
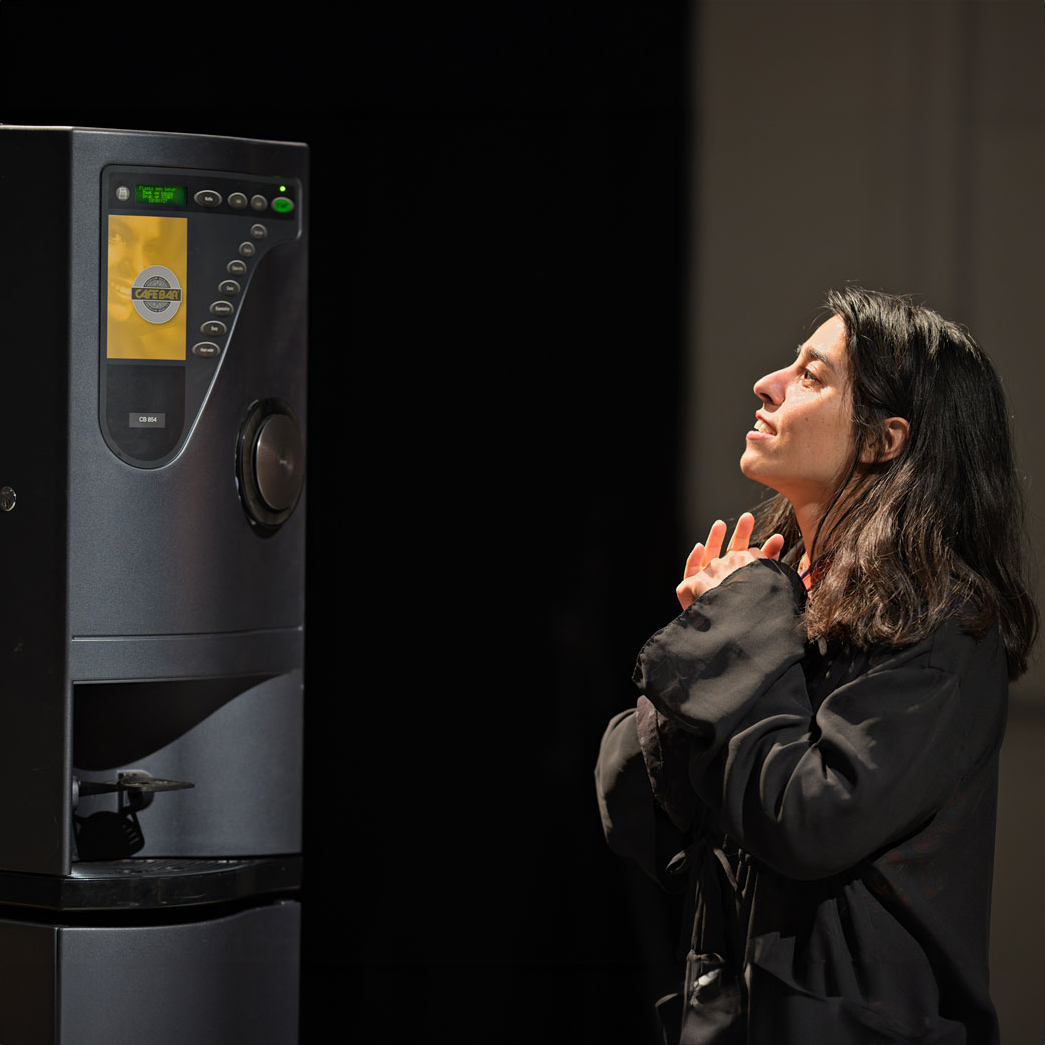
The installation invites curiosity, not just for a beverage, but for a conversation. Those not seeking coffee can still engage with the machine, discovering its witty observations and cynical commentary on its experiences and the environment it is exhibited in. It offers a light-hearted yet insightful reflection on the routines that define our lives, the desire for connection and our relationships with machines.
Supported by iii
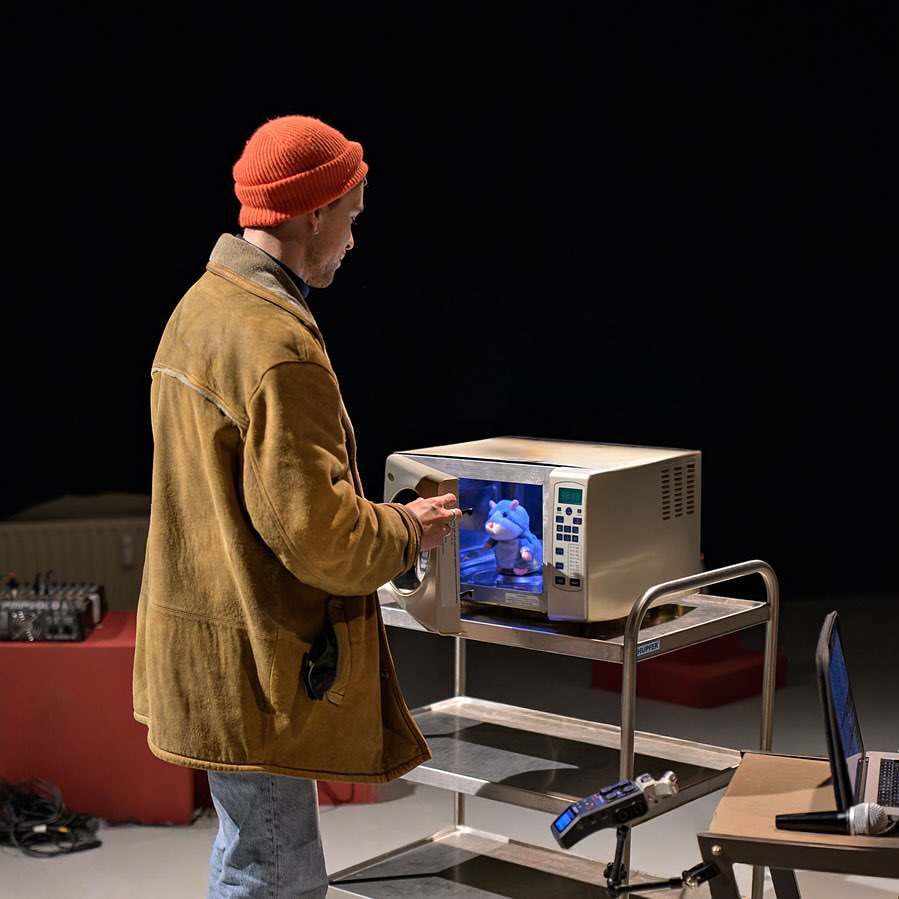
As visitors engage with the piece, they discover that the microwave possesses a unique ability: it can give a voice to any object placed within its interior. This transformative interaction allows for playful yet insightful conversations between the audience and their items, revealing the hidden narratives of the objects that silently serve us. Through its humorous and critical lens, "Microwave" prompts us to reflect on the roles these items play in our lives and the broader implications of our efficiency-obsessed culture, offering a mirror to our intricate relationship with the technologies that both empower and constrain us.
Supported by iii
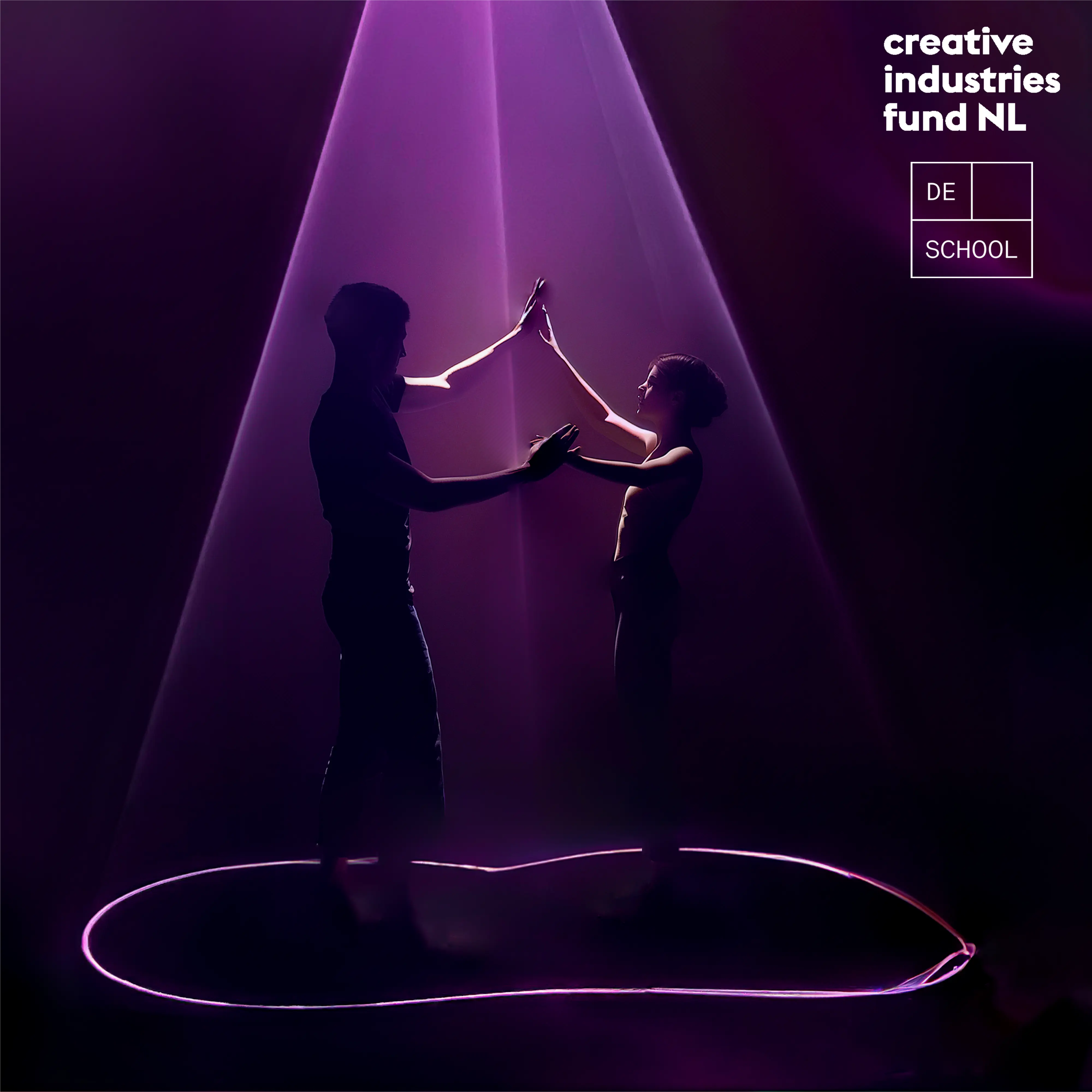
Developed in collaboration with Kawita ten Kate, during our residency at De School.
Sound design by Nawaz. Electromagnetic sensor development led by Marcel van der Bilt. Laser programming by Bram Snijders. Artistic guidance by Anne van der Weijden.
Supported by the Creative Industries Fund NL & De School
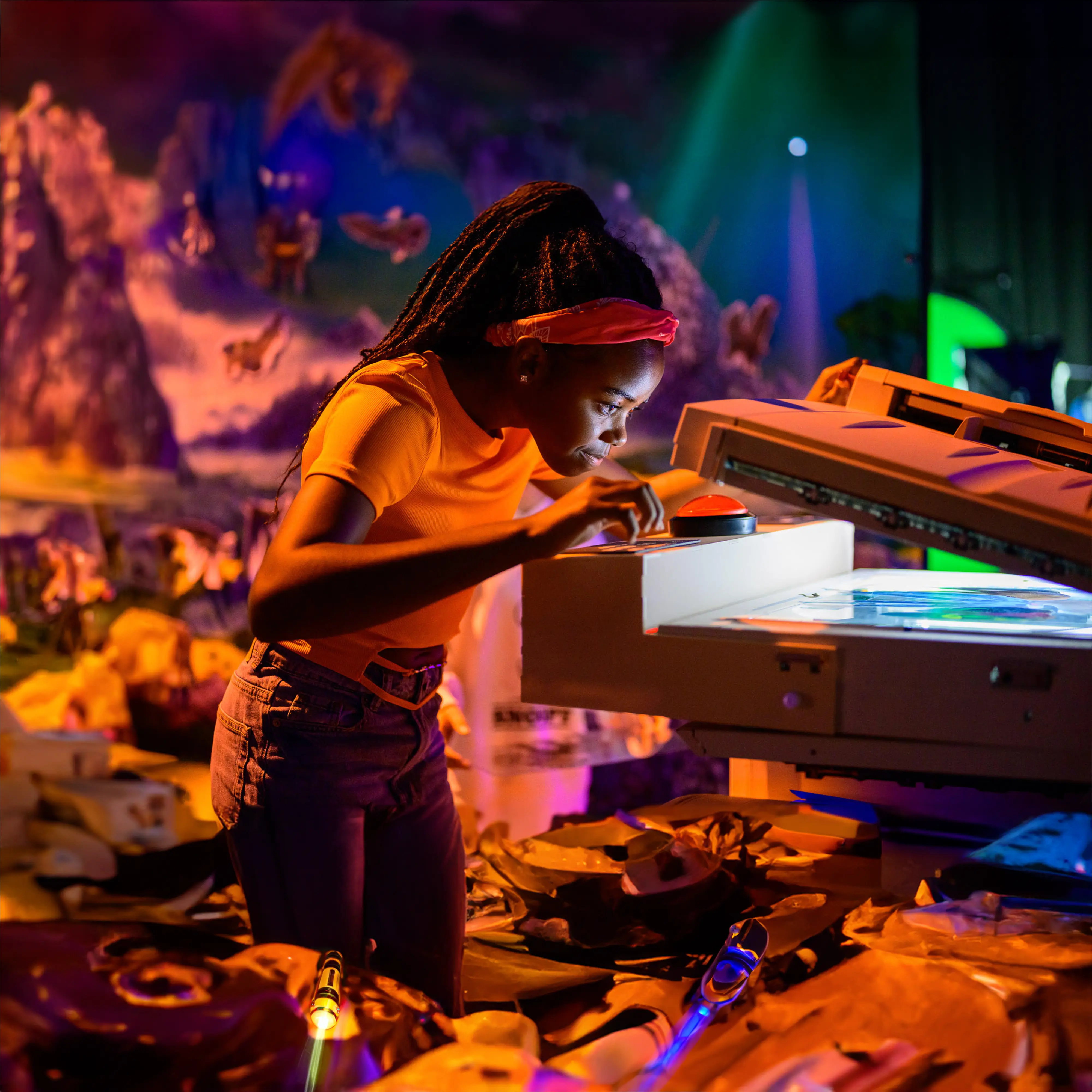
The idea behind this installation is to create a space where people can interact with a machine that is itself creative, raising awareness of the potential of machine learning as a tool for creativity. The machine is designed to inspire playfulness and be a fun and easy way for people to create art, without needing any prior experience or knowledge.
[Documentation]
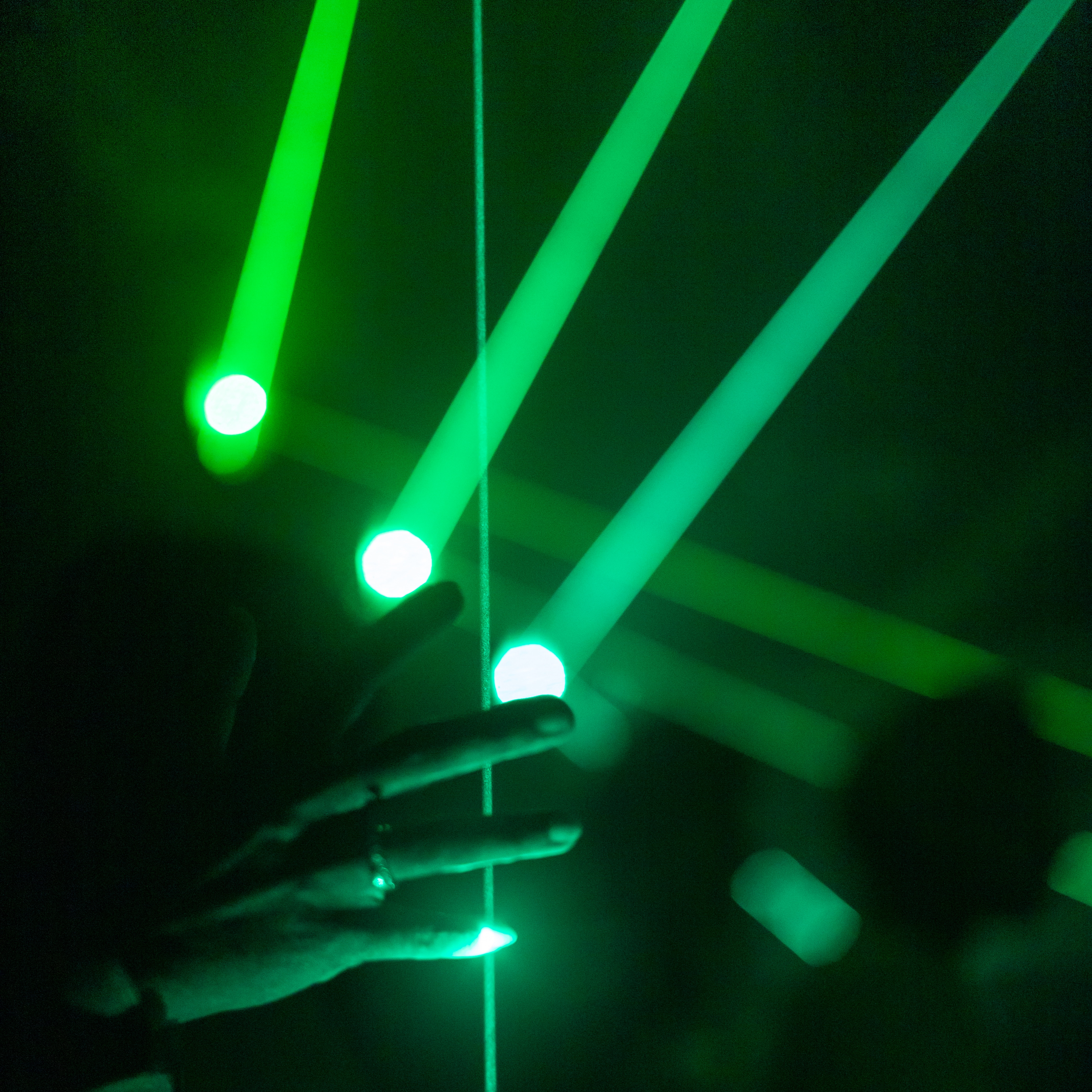
The physical installation consists of 7 individually moveable lasers combined with freely placeable mirrors to create site-specific patterns. Mounted next to every laser is a lidar sensor for measuring long- and fine-grained distance.
[Documentation]
Publications
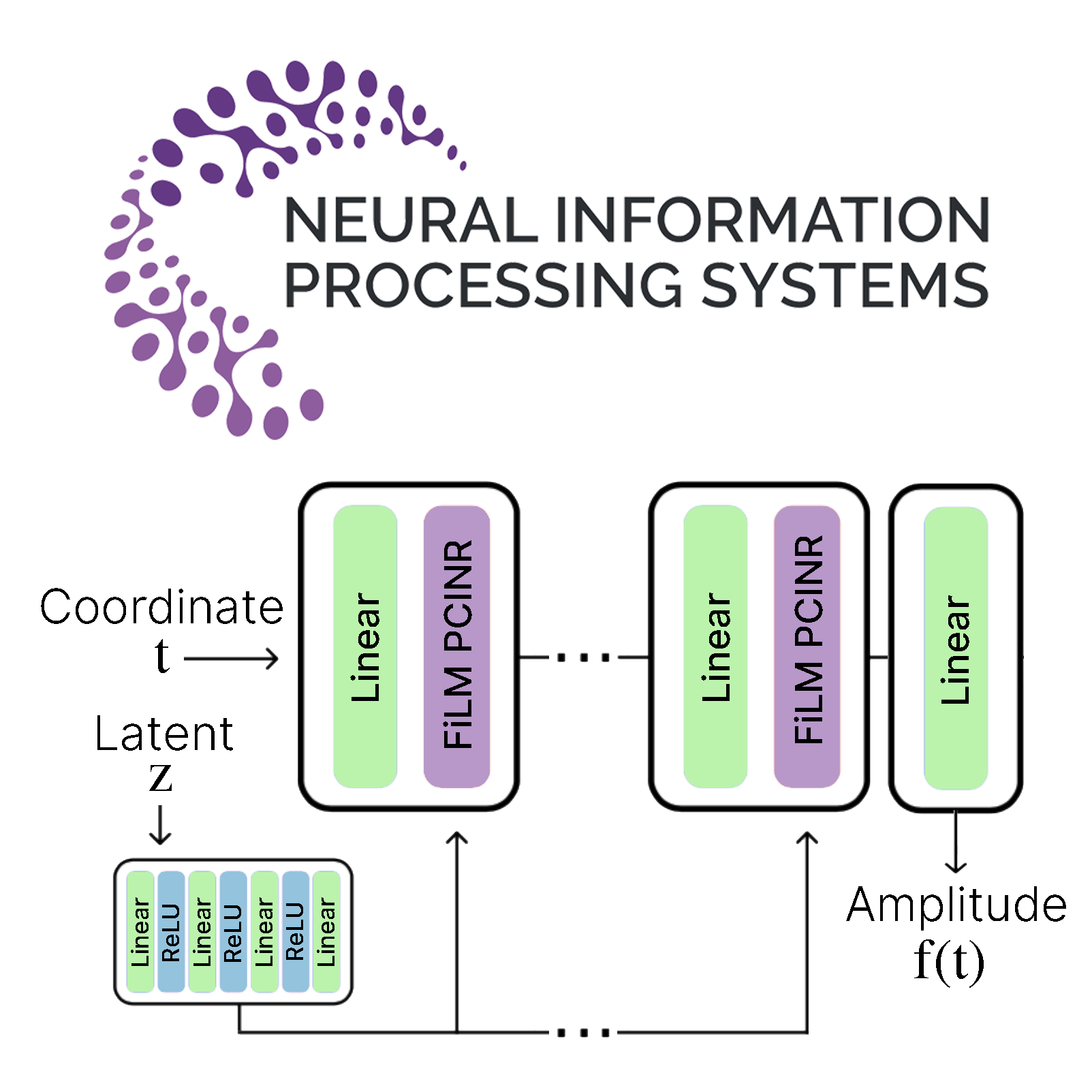
NeurIPS 2021, Machine Learning for Creativity and Design (Poster)
In this work, we aim to shed light on the potential of Conditional Implicit Neural Representations (CINRs) as lightweight backbones in generative frameworks for audio synthesis. Our experiments show that small Periodic Conditional INRs (PCINRs) learn faster and generally produce quantitatively better audio reconstructions than Transposed Convolutional Neural Networks with equal parameter counts. However, their performance is very sensitive to activation scaling hyperparameters. We validate noise presence can be minimized by applying standard weight regularization during training or decreasing the compositional depth of PCINRs, and suggest directions for future research.
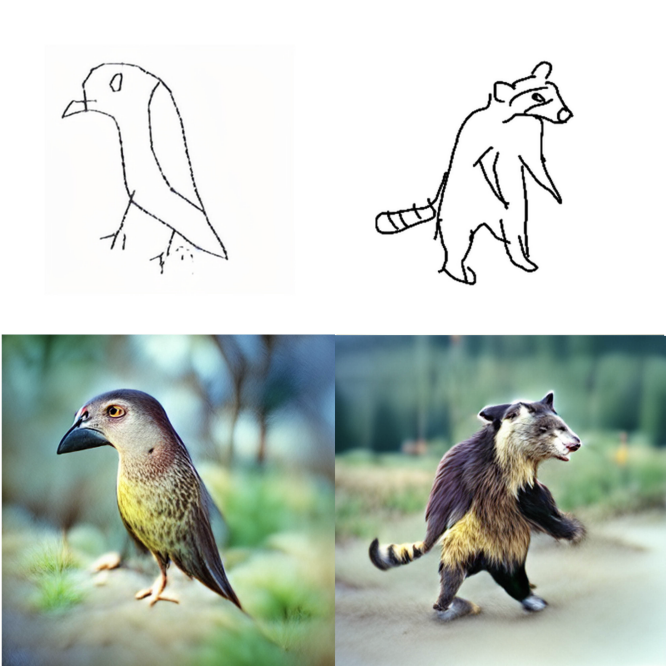
In this work, we propose and validate a framework to leverage language-image pretraining representations for training-free zero-shot sketch-to-image synthesis. Our approach for disentangling style and content entails a simple method consisting of elementary arithmetic assuming compositionality of information in representations of input sketches. Our results demonstrate that this approach is competitive with state-of-the-art instance-level open-domain sketch-to-image models, while only depending on pretrained off-the-shelf models and a fraction of the data.